Welcome to InsightsofAI.com, your trusted source for insights into Artificial Intelligence and Machine Learning. This guide explains Association Rule Mining, a machine learning technique that identifies patterns in datasets. By applying this method, businesses can uncover relationships between items and make data-driven decisions.
What is Association Rule Mining?
Association Rule Mining is a process used to identify meaningful relationships between items in a dataset. It is widely applied in:
- Customer Segmentation: Categorizing customers based on their purchase habits.
- Market Basket Analysis: Identifying products frequently bought together.
- Recommendation Systems: Suggesting items to users based on past behavior.
- Fraud Detection: Detecting unusual patterns in transactions.
Why is Association Rule Mining Useful?
Organizations collect vast amounts of transactional data daily. Association Rule Mining helps to extract insights that can:
- Improve customer satisfaction with personalized recommendations.
- Increase sales by identifying product combinations for cross-selling.
- Enhance inventory management by predicting product demand.
Key Concepts in Association Rule Mining
Support:
Measures how frequently an itemset appears in transactions

Confidence:
Indicates the likelihood of item B being purchased when item A is purchased.

Lift:
Evaluates the strength of a rule compared to random co-occurrence of items.
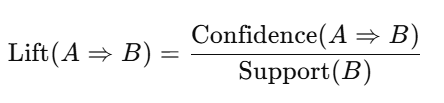
Popular Algorithms for Association Rule Mining
Apriori Algorithm
The Apriori Algorithm generates frequent itemsets by analyzing transactions. It works bottom-up, starting with individual items and extending to larger itemsets if they meet the minimum support threshold.
Advantages:
- Simple and easy to implement.
- Customizable thresholds for support and confidence.
Disadvantages:
- Computationally intensive for large datasets.
- Produces many redundant rules.
FP-Growth Algorithm
The FP-Growth Algorithm eliminates the need for candidate generation by compressing the dataset into a Frequent Pattern Tree (FP-Tree). Patterns are extracted directly from this compact structure.
Advantages:
- Faster than Apriori for large datasets.
- Requires less memory by compressing the data.
Disadvantages:
- Implementation can be complex.
- May use significant memory for imbalanced datasets.
Eclat Algorithm
The Eclat Algorithm calculates support using set intersections. It works in a depth-first search manner, making it suitable for dense datasets.
Advantages:
- Efficient for datasets with fewer unique items.
- Memory-friendly for dense datasets.
Disadvantages:
- Performance decreases with sparse datasets.
- Computationally heavy for large datasets.
Step-by-Step Implementation in Python
We applied the following algorithms using a real-world dataset:
- Dataset: Online Retail II
- GitHub Repository: Association Rule Algorithms in Python
Data Preprocessing
- Cleaned the dataset by removing missing values and duplicate records.
- Transformed the dataset into a format suitable for Association Rule Mining.
Exploratory Data Analysis (EDA)
- Analyzed transaction trends.
- Identified top-selling products and their patterns.
Algorithm Implementation
Apriori Algorithm Code:
from mlxtend.frequent_patterns import apriori, association_rules
frequent_itemsets = apriori(transactions, min_support=0.01, use_colnames=True)
rules = association_rules(frequent_itemsets, metric="lift", min_threshold=1.0)
FP-Growth Algorithm Code:
from mlxtend.frequent_patterns import fpgrowth
frequent_itemsets_fp = fpgrowth(transactions, min_support=0.01, use_colnames=True)
rules_fp = association_rules(frequent_itemsets_fp, metric="confidence", min_threshold=0.2)
Eclat Algorithm Code:
# Pseudo-code for Eclat
def eclat(transactions, min_support):
frequent_itemsets = {}
for itemset in combinations(unique_items, 2):
support = calculate_support(itemset)
if support >= min_support:
frequent_itemsets[itemset] = support
return frequent_itemsets
Results and Insights
- Frequent Itemsets: Discovered common product combinations purchased together.
- Association Rules: For example, “If a customer buys Gift Set, they are 80% likely to buy Mug.”
Performance Comparison:
- FP-Growth outperformed Apriori in speed and efficiency.
- Eclat performed well for dense datasets but struggled with sparse ones.
Practical Applications
- Retail: Grouping products frequently purchased together for marketing campaigns.
- E-commerce: Building recommendation systems for better customer retention.
- Healthcare: Identifying co-occurrences in medical diagnoses or prescriptions.
Additional Resources
- GitHub Repository: View Code
- Dataset: Online Retail II on Kaggle
Conclusion
Association Rule Mining is a critical tool for analyzing large transactional datasets. By applying Apriori, FP-Growth, and Eclat algorithms, businesses can uncover actionable patterns to optimize operations, improve sales, and enhance customer satisfaction. Start your journey into Association Rule Mining today!